We’ve all heard about how important data and data analytics are for the technology industry as a whole, but what does this mean specifically for Support organizations? Support has always had access to detailed customer data, but the ability to correctly capture, read, and apply the insights learned from this data, both directly and from support automation tools, is what can transform a Support organization into a pacesetter in the marketplace. That’s why the roles of data analyst and data scientists are becoming increasingly sought after in today’s Support organizations. To learn more about how Support orgs are investing in analytics experts, TSIA conducted a Quick Poll on the use of analytics for support services, which had some interesting results.
Which Functions Within an Organization Need Data Analytics?
There’s a common misconception that analytics are more important for Customer Success than they are for Support Services teams, but the truth is, they're just as important for Support. In fact, Support sits on most of the data that the Customer Success needs, so in reality, Support is actually enabling Customer Success with the data they need in order to fulfill their responsibilities.
That said, Analytics teams have rapidly become the heartbeat of many companies, driving momentous decisions in Support, Sales, Engineering, Marketing, and Customer Success. In our poll, we didn’t focus on the technical aspects of analytics capabilities, but rather on the Analytics personnel who are working directly with Support teams.
First, we asked our respondents about the access that their Support organization has to Analytics personnel. The great news is that almost 41% have access to a dedicated team of Analytics staff (including data analysts and data scientists), and close to 30% have access to a shared team of Analytics resources. Unfortunately, 30% of our respondents shared that they don’t have any access to these resources.

We asked those 30% who said they don’t have access to analytics whether they had plans in the next 6 months to create an Analytics team. Of that group, 29% said “no”, 29% said “yes”, but interestingly, 41% said “other”. When we probed into this response, we found such answers as:
- “Yes, but more like 12 months from now.”
- “We’re still deliberating if it’s a need that we have.”
- “We would love to, but there’s no budget.”
- “Our analytics are done by our leadership team, and we aren’t sure we need more than what are leaders are already doing.”
- “As a combined organization, we’re looking at this capability, but we’re not sure if Support will get access to the analytics personnel.”
If any of these describes what’s happening within your organization, you need to do whatever you can to move this “other” into a “yes”, because analytics for Support is going to be a critical element for future success from here and going forward.
What Size of Analytics Team Do We Need?
Before I answer this question, I should provide some context around the size of companies that responded to this Quick Poll, so here’s a snapshot:
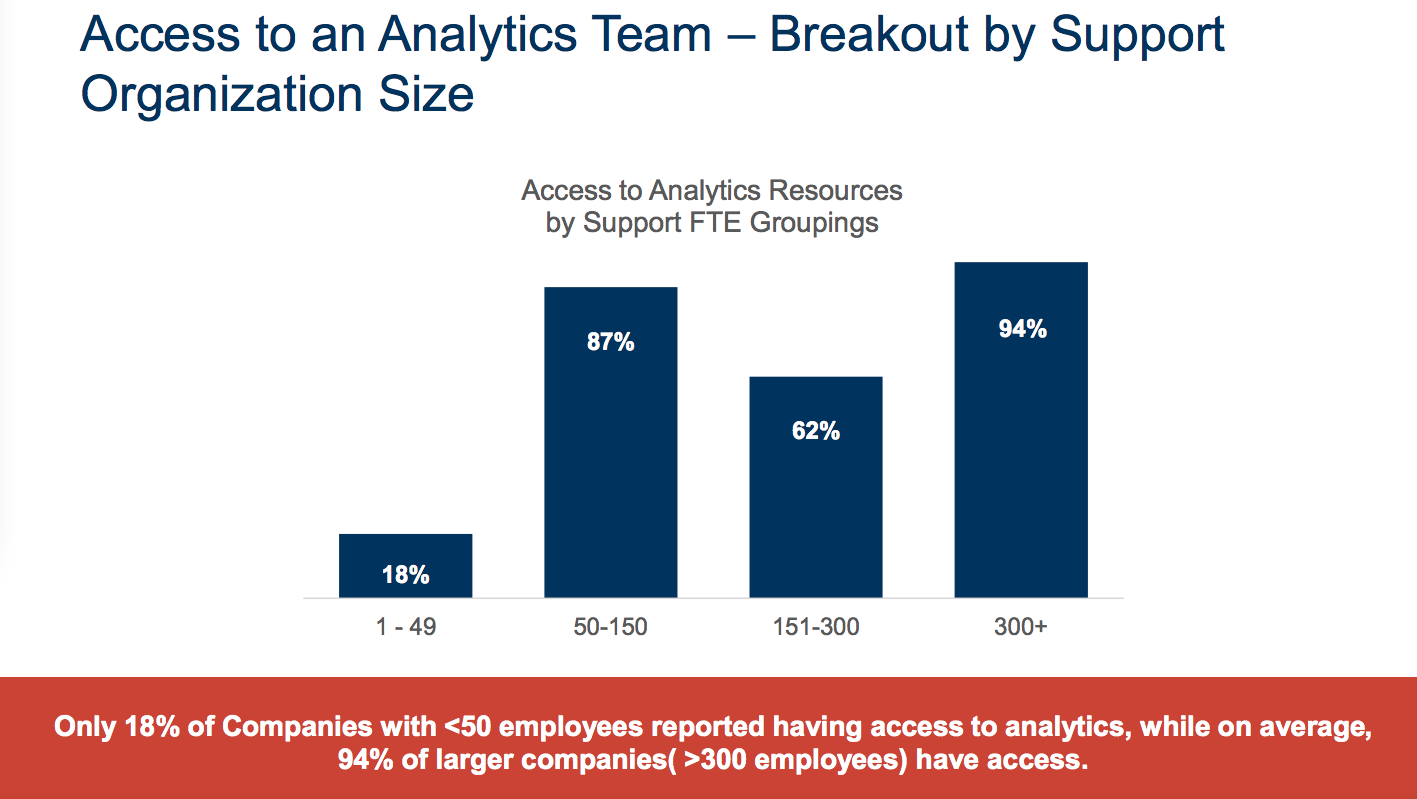
Shockingly, only 18% of companies with under 50 employees reported having access to analytics, while on average, 94% of larger companies (over 300 employees) have access. This ratio isn’t uncommon to see, and further solidifies the idea that Analytics is now being viewed as the heartbeat of modern organizations.
Smaller organizations have approximately 1-2 people, either in a dedicated or shared Analytics role. However, across the board the average number of Analytics employees is 5, with as many as 16 being employed by organizations with over 1k employees.
What Kind of Data Will the Analytics Team Work With?
Support organizations have always been very good at collecting hundreds and hundreds of metrics, but the number one inquiry I receive from TSIA Support Services members is always around what to measure, how to measure it, who to share it with, and how to apply this valuable data to improving their performance.
When we look at analytics, we can break it down into “basic” and “advanced.” Keep in mind that assigning the word “basic” doesn’t mean that this data isn’t important, as it includes such metrics as basic reporting and KPI tracking, which are very valuable to Support organizations.
Advanced analytics are a great thing to have, and 75% of our respondents have said this capability has drastically improved their support services overall. The good news is that regardless of whether you have a dedicated Analytics team that’s just for Support or you’re sharing with the rest of your organization, you will reap the same level of benefit, such as predictive NPS scoring, driving less surveys (and therefore mitigating survey fatigue), reducing costs, and even using this data to predict CSAT and NPS.
Examples of Why Your Support Services Organization Needs Customer Support Analytics: Case Studies
At TSIA’s last Technology Services World conference, I heard the phrase, “Any Support organization who doesn’t have data analytics staff is a Support organization that will be in trouble in the future,” and I couldn’t agree more. Here are some examples of real TSIA members who have received value from investing in their Analytics team and related capabilities.
Support Services Example: One TSIA member was able to use data analytics to identify a root cause of a variety of issues they were having, which allowed them to significantly reduce their case abandon rate and increase their average speed of answer by 50% year over year without increasing their staffing. The CSAT that they saw increases in YoY tied directly to the analysis of verbal customer feedback and CSAT data they collected. As a result, they now receive ~25,000 survey responses per year, and their team now does analytics around it to identify dissatisfaction drivers.
Relationship Example: Another member added a customer health score (CHS) dashboard to their Renewals team and Cloud Delivery managers. This dashboard provides access to the health scores of their Maintenance and Cloud & Subscription customers, and is a measure of their relationship with their customers, including how well the customer is leveraging their products and services. They found that the lower the score, the higher the opportunity there was for them to start/restart a relationship (Land and Renew in the context of TSIA’s LAER customer engagement model).
Channel Example: One TSIA member implemented a chat option as a customer engagement channel based on assessing which products had a high volume of cases with a severity level of 3-4, as well as which products had high first-day close rates.
CSAT and Headcount Example: The use of predictive analytics showed this TSIA member their CSAT and headcount needs over the next 3 years, which influenced new product business case approvals.
Smart Tip: Embrace Data-Driven Decision Making
Making smart, informed decisions is more crucial than ever. Leveraging TSIA’s in-depth insights and data-driven frameworks can help you navigate industry shifts confidently. Remember, in a world driven by artificial intelligence and digital transformation, the key to sustained success lies in making strategic decisions informed by reliable data, ensuring your role as a leader in your industry.